Safety Monitoring & Forecasting
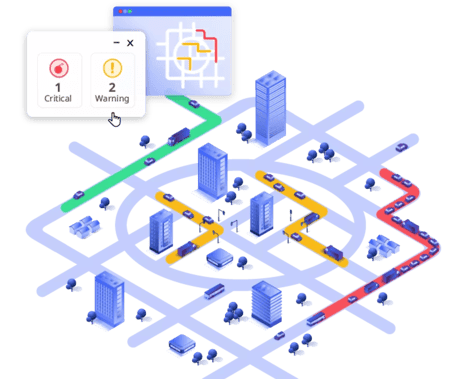
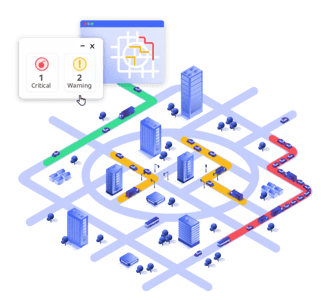
Safety monitoring and forecasting in transportation involve the use of various techniques and technologies to analyze and predict safety-related events, incidents, or conditions in transportation systems. The aim is to enhance overall safety, reduce accidents, and mitigate risks for all road users, including drivers, pedestrians, and cyclists. Here's an overview of safety monitoring and forecasting in transportation:
1. Data Collection and Analysis:
Data forms the foundation of safety monitoring and forecasting. Various data sources are utilized, including traffic flow data, weather conditions, accident reports, vehicle characteristics, road infrastructure, and more.
Advanced data analytics techniques, including machine learning and statistical analysis, are applied to process and extract meaningful insights from the collected data.
2. Safety Performance Indicators (SPIs):
SPIs are used to measure and monitor safety levels in transportation systems. These indicators may include metrics such as accident rates, fatality rates, injury severity, and near misses.
Real-time monitoring and analysis of SPIs provide valuable information to identify areas with high safety risks and prioritize interventions accordingly.
3. Predictive Modeling:
Predictive modeling involves the development of mathematical or computational models to forecast future safety events based on historical data and other relevant factors.
Machine learning algorithms are commonly used to build predictive models that can forecast accident hotspots, vulnerable road segments, or times of high risk.
4. Risk Assessment:
Risk assessment involves evaluating potential risks associated with various transportation factors, including road conditions, traffic volume, weather, and driver behavior.
Quantitative risk assessment methodologies help in identifying potential safety hazards and determining appropriate risk mitigation measures.
5. Traffic Management and Control:
Dynamic traffic management systems are employed to monitor traffic flow, detect congestion, and manage traffic signals to improve safety and reduce the likelihood of accidents.
Intelligent transportation systems (ITS) play a crucial role in managing traffic and enhancing safety through technologies such as adaptive traffic signals, variable message signs, and automated incident detection.
6. Emerging Technologies:
Advanced technologies like connected and autonomous vehicles (CAVs), vehicle-to-infrastructure (V2I), and vehicle-to-vehicle (V2V) communication systems contribute to improved safety by enabling real-time information sharing and collision avoidance.
7. Policy and Regulation:
Governments and transportation authorities play a vital role in formulating and enforcing policies and regulations aimed at enhancing safety in transportation.
These policies may encompass speed limits, seat belt laws, driving under the influence (DUI) laws, and initiatives promoting safe driving practices.
8. Public Awareness and Education:
Public education campaigns and initiatives are crucial in promoting safe driving habits, reducing distractions, and raising awareness about the importance of road safety.
By leveraging data, advanced technologies, predictive modeling, and policy interventions, safety monitoring and forecasting in transportation contribute to creating safer road environments and reducing accidents and fatalities.
Now, Our products in Safety Monitoring & Forecasting:
This web application provides forecasts of crash risk in the transport network based on different months, days of the week, and time intervals. It takes into account variables such as land use, geometric design, and weather, which significantly influence risk on specific segments. Users ranging from public transport companies like school buses to taxi and freight companies can utilize this application to select routes with minimal risk.
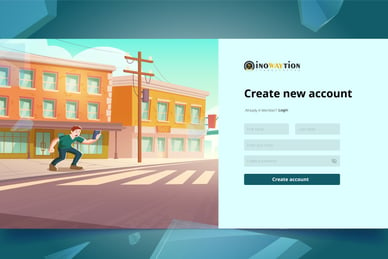
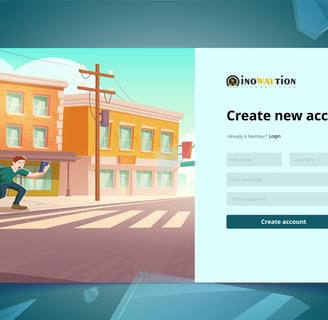
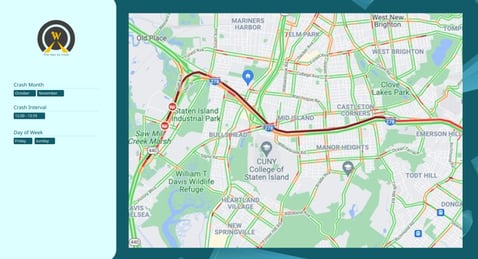
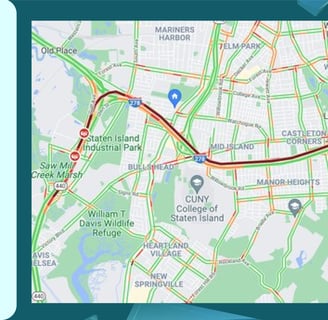
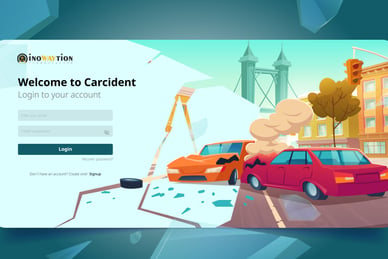
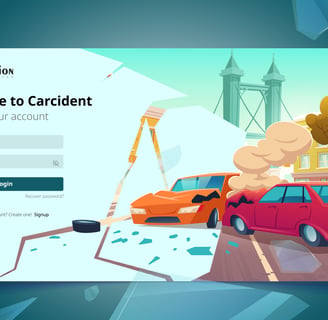
Safety Risk Map Application
This study focuses on traffic safety forecasting for road segments, with a significant emphasis on rural networks often overlooked in previous research. Rural roads pose a higher risk of severe accidents. The research aims to analyze traffic accidents in these areas, identify factors influencing crash frequency, and propose a spatial-temporal crash risk mapping framework to prioritize high-risk segments on different days. Data from the Sydney province is utilized, employing crash frequency data collected daily and at a spatial resolution of 1500 meters from loop detectors.
The study categorizes influential factors into four groups: traffic parameters (e.g., traffic flow, speed, time headway), road characteristics (e.g., road type, number of lanes), weather data (e.g., daily rainfall, snow depth, temperature), and calendar variables (e.g., day of the week, public holidays, month, year), used for model calibration. Three decision tree algorithms—Decision Tree (DT), Random Forest (RF), and eXtreme Gradient Boosting (XGBoost)—are utilized to predict crash frequency.
Results highlight that XGBoost effectively explains and interprets factors affecting crash frequency, while RF excels in trend detection and crash frequency forecasting. Notably, traffic flow rate, road type, crash year, and wind speed emerge as the most influential variables in predicting crash frequency on rural roads. Accurate forecasting of high and medium-risk segments on specific days in rural networks is crucial for effective safety management planning. This risk assessment is highly dependent on real traffic data, weather forecasts, and road geometric characteristics. The study successfully predicts seventy percent of high and medium-risk segment-days for the case study.



INOWAYTION PTY LTD, Sydney Knowledge Hub, Level 2, Merewether Building H04, University of Sydney, NSW 2006


+61 4 51870400
Contact Us

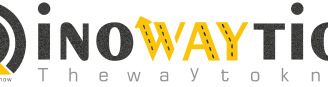
Inowaytion is an agile and specialized company in the field of logistics and transportation, which has created a new development in the road industry by using experts in the field of transportation, artificial intelligence and computers.

