Traffic Management & Traffic forecasting
Traffic management and traffic forecasting systems are crucial components of transport planning and management. They play a significant role in ensuring efficient and safe transportation in cities and regions. Let's delve into each of these systems:
1. Traffic Management:
Traffic management involves the control and optimization of traffic flow on roadways and transportation networks to ensure smooth and safe travel for vehicles and pedestrians. Here are some key aspects of traffic management:
· Traffic Signals: Traffic signals at intersections are one of the most common tools for managing traffic. They control the flow of vehicles by regulating when different directions of traffic can proceed.
· Traffic Signs and Markings: Signs and road markings provide essential information to drivers, such as speed limits, warnings of hazards, and directions. Proper signage helps in managing traffic by guiding drivers.
· Traffic Enforcement: Law enforcement agencies monitor and enforce traffic rules to ensure compliance and safety. This includes issuing tickets for violations like speeding, running red lights, or reckless driving.
· Traffic Control Devices: These include tools like barriers, cones, and road closures used to manage traffic in specific areas, such as construction zones or accident scenes.
· Public Transportation: Encouraging the use of public transportation can help reduce traffic congestion. Effective public transit systems are a vital part of traffic management in urban areas.
· Traffic Data Collection: The use of sensors, cameras, and other data collection methods helps traffic managers monitor real-time traffic conditions and make adjustments as needed.
2. Traffic Forecasting System:
· Data Collection: These systems gather data from various sources, including traffic counters, sensors, GPS devices, and historical traffic data. They also consider factors like population growth, economic development, and urban expansion.
· Traffic Modeling: Using collected data, traffic forecasting systems create mathematical models to simulate traffic patterns and predict future conditions. These models can be highly sophisticated and account for various variables.
· Scenario Analysis: Traffic forecasting systems can simulate different scenarios, such as the impact of new road construction, changes in public transportation, or the effects of special events, on traffic flow.
· Planning and Decision-Making: Planners and policymakers use the forecasts generated by these systems to make informed decisions about transportation infrastructure investments, road expansion projects, and traffic management strategies.
· Congestion Mitigation: By predicting areas where traffic congestion is likely to occur in the future, authorities can take proactive measures to mitigate congestion, such as implementing traffic management solutions or improving public transportation options.
Traffic forecasting is the process of predicting future traffic patterns and volumes based on historical data, current trends, and various influencing factors. These systems are essential for urban planning, infrastructure development, and optimizing transportation networks. Here's how traffic forecasting systems work:
In summary, traffic management focuses on the day-to-day control and regulation of traffic to ensure safety and efficiency, while traffic forecasting systems help cities and regions plan for the future by predicting traffic patterns and guiding infrastructure and policy decisions. Both are essential for maintaining effective transportation systems in urban areas.
Now, Our product in traffic management:
The goal of short-term traffic prediction is to provide pre-travel information to travelers. This study utilizes machine learning methods, including long short-term memory (LSTM), random forest (RF), support vector machine (SVM), and K-nearest neighbors (KNN), to forecast traffic states categorized as A to C for rural road segments. Due to the irregular temporal variations in rural road traffic, the performance of these algorithms fluctuates across different time intervals. To enhance prediction accuracy for each time interval and segment, various ensemble methods, such as voting and the ordinal logit (OL) model, are employed to combine predictions from the four machine learning algorithms. The rural road traffic data serves as a case study to illustrate the implementation.
Given the multiple influential features affecting traffic state, a genetic algorithm (GA) identifies 25 out of 32 key features crucial for model fitness. The study demonstrates that the OL model, functioning as an ensemble learning model, surpasses individual machine learning models, achieving an accuracy of 80.03 percent. Specifically, the OL model attains the highest balanced accuracies for predicting traffic states A, B, and C at 89 percent, 73.4 percent, and 58.5 percent, respectively.



INOWAYTION PTY LTD, Sydney Knowledge Hub, Level 2, Merewether Building H04, University of Sydney, NSW 2006


+61 4 51870400
Contact Us

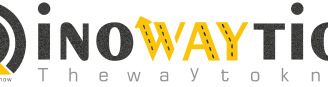
Inowaytion is an agile and specialized company in the field of logistics and transportation, which has created a new development in the road industry by using experts in the field of transportation, artificial intelligence and computers.

